knowledge, the teacher is able to provide the neural network with a desired responsefor that training vector. Indeed, the desired response represents the "optimum" ac-tion to be performed by the neural network. The network parameters are adjustedunder the combined influence of the training vector and the error signal. The errorsignal is defined as the difference between the desired response and the actual re-sponse of the network. This adjustment is carried out iteratively in a step-by-stepfashion with the aim of eventually making the neural network emulate the teacher;the emulation is presumed to be optimum in some statistical sense. In this way,knowledge of the environment available to the teacher is transferred to the neuralnetwork through training and stored in the form of"fixed" synaptic weights, repre-senting long-term memory. When this condition is reached, we may then dispensewith the teacher and let the neural network deal with the environment completelyby itself.
The form of supervised learning we have just described is the basis of error-correction learning. From Fig. 24, we see that the supervised-learning process con-stitutes a closed-loop feedback system, but the unknown environment is outside theloop. As a performance measure for the system, we may think in terms of the mean-square error, or the sum of squared errors over the training sample, defined as a func-tion of the free parameters (i.e., synaptic weights) of the system. This function maybe visualized as a multidimensional error-performance surface, or simply error surface,with the free paiameters as coordinates.The true error surface is averaged over allpossible input-output examples. Any given operation of the system under theteachers supervision is represented as a point on the error surface. For the system toimprove performance over time and therefore learn from the teacher, the operatingpoint has to move down successively toward a minimum point of the error surface;the minimum point may be a local minimum or a global minimum. A supervisedlearning system is able to do this with the useful information it has about the gradient of the error surface corresponding to the current behavior of the system.
《神经网络与机器学习(英文版·第3版)》((加)海金(Haykin
来源:互联网 发布日期:2011-09-20 18:43:17 浏览:22930次
导读:神经网络与机器学习(英文版·第3版)作者:(加)海金(Haykin,S)机械工业出版社出版,京东人工智能图书网购,折扣超低。...
下一篇:没有了...
相关内容
- 中信建投:关注5G-A、卫星通信、FTTR等新一代通信基础设施建设
- AI+5G推动大模型基础设施化 为全面智能社会奠基
- 爱立信中国区总裁方迎:构建新质网络 推动5G下半场实现卓越价值
- 尚网网络CEO朱立华:WiFi技术有助解决5G峰值流量问题
- 爱立信中国区总裁方迎:5G发展仍面临两大挑战,“新质网络”将创造“新质价值”
- 推进5G向5G-A升级,券商:一体化算力体系新基建有望夯实
- 中国科学院院士尹浩:5G商用遍布全球,仍需解决安全保障问题
- 苹果自研5G基带细节曝光:3年3款芯片,全面替代高通
- 中国广电曾庆军:5G NR广播扩展到卫星覆盖,国产芯片的车载音视频系统等均可预载功能
- 当5G-A与AI融合,联接网络底座需不断创新与演进
- 高通孟樸:以5G和AI正在加速推动多个行业的数字化转型和创新发展
- 国家数据局:有序推进5G网络向5G-A升级演进 全面推进6G网络技术研发创新
- 如何买到便宜大碗的 5G 套餐?聊聊你也许不知道的 CPE、MiFi 和物联卡
- 5G网络:向未来演进,开启全面智能新时代
- 天翼智库饶少阳:AI+5G融合助推高阶广泛智能落地
- 印度中产“新宠”:5G网络成生活必需,民众愿意为高速网络买单
- 5G规模化应用时间表确定 5G-A支撑低空经济加速落地 通信业增长空间进一步打开|行业观察
- 通讯观察|大模型赋能5G 通讯行业“AI+”大幕已拉开
- 高通孟樸:5G和AI的融合为新型工业制造带来智能化飞跃
- 多地加速手机补贴政策落地,5G换机窗口期还有多久?
AiLab云推荐
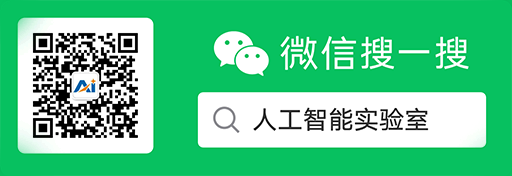
最新资讯
本月热点
- 如何买到便宜大碗的 5G 套餐?聊聊你也许不知道的 CPE、MiFi 和物联卡
- 爱立信中国区总裁方迎:5G发展仍面临两大挑战,“新质网络”将创造“新质价值”
- 东南亚多国邀中企合作开发5G网络,泰总理:愿同中方在多领域加强合作
- 从数字化到智能化,探访中国首个五星5G工厂 中兴通讯副总裁陆平:5G革新了生产方式
- 苹果自研5G基带细节曝光:3年3款芯片,全面替代高通
- 5G网络:向未来演进,开启全面智能新时代
- 中国科学院院士尹浩:5G商用遍布全球,仍需解决安全保障问题
- 天翼智库饶少阳:AI+5G融合助推高阶广泛智能落地
- 中国广电曾庆军:5G NR广播扩展到卫星覆盖,国产芯片的车载音视频系统等均可预载功能
- 5G规模化应用时间表确定 5G-A支撑低空经济加速落地 通信业增长空间进一步打开|行业观察
热门排行
-
当海外华文媒体遇见中国首座5G煤矿:数智转型就在眼前
阅读量:8179
-
如何买到便宜大碗的 5G 套餐?聊聊你也许不知道的 CPE、MiFi 和物联卡
阅读量:4228
-
5G-A商用加速:北京运营商齐发力,创新应用场景欣欣向荣
阅读量:4140
-
美版苹果 iPhone 16 Pro 实测:5G下载超400 Mbps、平均增幅23.7%
阅读量:3403
-
消息称国内“通信大厂开始搞 5G SOC”
阅读量:3194
-
精彩回顾 | 5G-A与AI融合产业论坛行业大咖观点
阅读量:3183